使用TensorFlow进行单变量时间序列预测¶
Info
作者:Vincent,发布于2022-05-14,阅读时间:约2分钟,微信公众号文章链接:
1 前言¶
本文将使用TensorFlow解决时间序列预测的问题,TensorFlow官网有一个非常详尽但冗长的教程,所以本文将剥茧抽丝,用通俗易懂的办法过一遍单变量时间序列预测最核心的内容。
2 比特币价格数据集¶
2.1 获取数据¶
本文使用比特币历史价格数据(2013年10月至2021年5月)进行预测,请注意本文不构成投资建议!
!wget https://raw.githubusercontent.com/mrdbourke/tensorflow-deep-learning/main/extras/BTC_USD_2013-10-01_2021-05-18-CoinDesk.csv
import pandas as pd
import matplotlib.pyplot as plt
import os
import tensorflow as tf
from tensorflow.keras as layers
df = pd.read_csv("/content/BTC_USD_2013-10-01_2021-05-18-CoinDesk.csv",
parse_dates=["Date"],
index_col=["Date"]) # Parse the date column
df.tail()
返回为:
Date | Currency | Closing Price (USD) | 24h Open (USD) | 24h High (USD) | 24h Low (USD) |
---|---|---|---|---|---|
2021-05-14 | BTC | 49764.132082 | 49596.778891 | 51448.798576 | 46294.720180 |
2021-05-15 | BTC | 50032.693137 | 49717.354353 | 51578.312545 | 48944.346536 |
2021-05-16 | BTC | 47885.625255 | 49926.035067 | 50690.802950 | 47005.102292 |
2021-05-17 | BTC | 45604.615754 | 46805.537852 | 49670.414174 | 43868.638969 |
2021-05-18 | BTC | 43144.471291 | 46439.336570 | 46622.853437 | 42102.346430 |
在此取收盘价进行预测:
bitcoin_prices = pd.DataFrame(df["Closing Price (USD)"]).rename({"Closing Price (USD)":"Price"},axis=1)
bitcoin_prices.head()
Date | Price |
---|---|
2013-10-01 | 123.65499 |
2013-10-02 | 125.45500 |
2013-10-03 | 108.58483 |
2013-10-04 | 118.67466 |
2013-10-05 | 121.33866 |
通过图表查看比特币价格走势:
plt.plot(bitcoin_prices["Price"])
plt.ylabel("Bitcoin price")
plt.xlabel("Date")
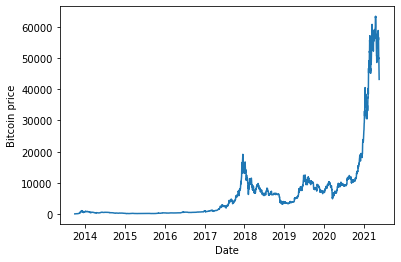
2.2 制作时间窗口¶
预期的数据格式为[0,1,2,3,4,5,6] -> [7]
,即使用过去七天的价格预测接下来一天的价格。在此,使用TensorFlow提供的timeseries_dataset_from_array API进行窗口划分。
timesteps = bitcoin_prices.index.to_numpy()
prices = bitcoin_prices["Price"].to_numpy()
HORIZON = 1 # 预测下一天的价格
WINDOW_SIZE = 7 # 基于过去7天的价格
input_data = prices[:-HORIZON]
targets = prices[WINDOW_SIZE:]
dataset = tf.keras.preprocessing.timeseries_dataset_from_array(
input_data, targets, sequence_length=WINDOW_SIZE)
for batch in dataset:
inputs, targets = batch
assert np.array_equal(inputs[0], prices[:WINDOW_SIZE])
assert np.array_equal(targets[0], prices[WINDOW_SIZE])
print(f"First Input:{inputs[0]}, Target:{targets[0]}")
print(f"Second Input:{inputs[1]}, Target:{targets[1]}")
break
返回如下,数据正确地转化成了预期的格式。
First Input:[123.65499 125.455 108.58483 118.67466 121.33866 120.65533 121.795 ], Target:123.033
Second Input:[125.455 108.58483 118.67466 121.33866 120.65533 121.795 123.033 ], Target:124.049
2.3 分割数据¶
使用时间来划分训练集和验证集,仅做示例故不留测试集,实际中可按需操作。
# tf.keras.preprocessing.timeseries_dataset_from_array返回的是batched dataset,所以先unbatch,方便分割数据
dataset = dataset.unbatch()
test_split = 0.2
split_index = int(len(list(dataset)) * (1-test_split))
# 分割完之后再分Batch,增加一维,不然无法满足模型数据维度要求
train_dataset = dataset.take(split_index).batch(batch_size=32)
test_dataset = dataset.skip(split_index).batch(batch_size=32)
3 建模¶
本文不追求极致的预测准确率,因此仅使用全连接层构建模型,代码如下:
tf.random.set_seed(42)
tf.keras.backend.clear_session()
# 1. 构建模型
model = tf.keras.models.Sequential(
[
layers.Input(WINDOW_SIZE),
layers.Dense(128, activation="relu"),
layers.Dense(HORIZON, activation="linear")
]
, name="model_dense_base")
# 2. 编译模型
model.compile(loss='mae',
optimizer=tf.keras.optimizers.Adam(),
metrics=['mae'])
# 创建callback把表现最好的模型checkpoint存下来
def create_model_checkpoint(model_name, save_path="model_checkpoint"):
return tf.keras.callbacks.ModelCheckpoint(filepath=os.path.join(save_path, model_name),
verbose=0,
save_best_only=True)
# 3. 训练模型
model.fit( train_dataset,
epochs=100,
verbose=0,
validation_data=test_dataset,
callbacks=[create_model_checkpoint(model_name=model.name)]
)
将表现最好的模型加载回来做评估:
model = tf.keras.models.load_model("model_checkpoint/model_dense_base")
model.evaluate(test_dataset)
返回如下,由平均绝对误差(MAE)可见预测的价格平均和真实价格相差700多美元。
18/18 [==============================] - 1s 6ms/step - loss: 759.4327 - mae: 759.4327
[759.4326782226562, 759.4326782226562]
考虑到模型结构十分简单,结果有提升空间也在预期之内,根据本流程进行优化,完全可以预测得更准确。
4 总结¶
本文对单变量时间序列预测任务做了一个基准,其中TensorFlow的tf.keras.preprocessing.timeseries_dataset_from_array
API简化了许多处理时间窗口的工作,之后将继续对TensorFlow预测时间序列的任务进行讨论。
5 相关阅读资料¶
%20-%20Tail%20Pic.png)
Viewed times